Parking Slot Detection Opencv
As the spots are well defined and assuming camera is fixed, you could use opencv background subtraction with sufficient amount of history to detect if there is an object in a particular parking spot. Look at this opencv tutorial for more information. I am trying to use opencv to automatically find and locate all parking spots in an empty parking lot. Currently, I have a code that thresholds the image, applies canny edge detection, and then uses probabilistic hough lines to find the lines that mark each parking spot. The program then draws the lines and the points that make up the lines.
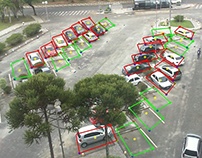
Prev Tutorial:Laplace Operator
Requires OpenCV 3.0. Requires python3. To run the project run the following comand. Python main.py -image images/prototype1re.png. Automatic smart parking system is emerging field and attracted computer vision researchers to contribute in this arena of technology. In this paper, we have presented a vision based smart parking framework to assist the drivers in efficiently finding suitable parking slot and reserve it. (2013) Parking Lot Occupancy Detection Using Computational Fluid Dynamics. In: Burduk R., Jackowski K., Kurzynski M., Wozniak M., Zolnierek A. (eds) Proceedings of the 8th International Conference on Computer Recognition Systems CORES 2013.
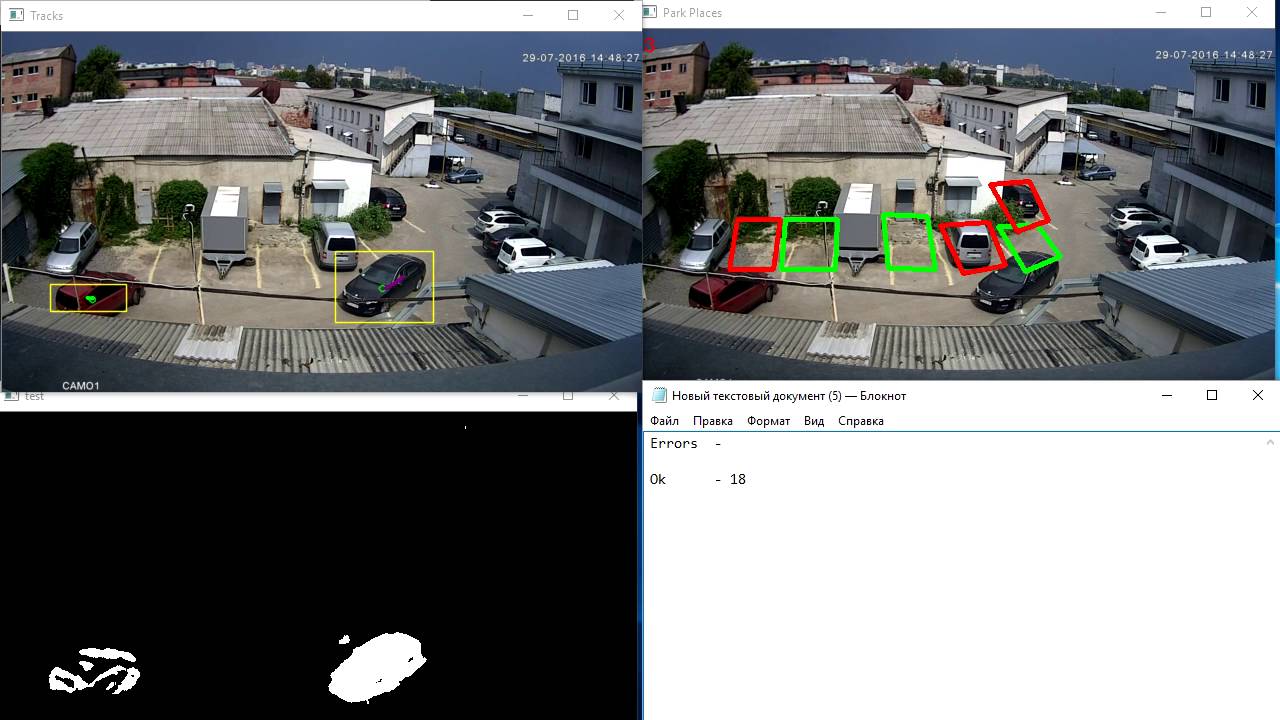
Next Tutorial:Hough Line Transform
Original author | Ana Huamán |
Compatibility | OpenCV >= 3.0 |
Goal
In this tutorial you will learn how to:
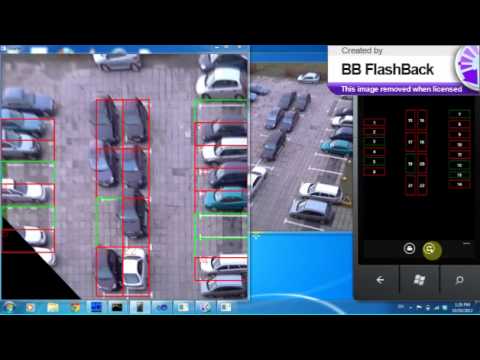
- Use the OpenCV function cv::Canny to implement the Canny Edge Detector.
Theory
The Canny Edge detector[36] was developed by John F. Canny in 1986. Also known to many as the optimal detector, the Canny algorithm aims to satisfy three main criteria:
Parking Slot Detection Opencv Software

- Low error rate: Meaning a good detection of only existent edges.
- Good localization: The distance between edge pixels detected and real edge pixels have to be minimized.
- Minimal response: Only one detector response per edge.
Steps
Filter out any noise. The Gaussian filter is used for this purpose. An example of a Gaussian kernel of (size = 5) that might be used is shown below:
[K = dfrac{1}{159}begin{bmatrix} 2 & 4 & 5 & 4 & 2 4 & 9 & 12 & 9 & 4 5 & 12 & 15 & 12 & 5 4 & 9 & 12 & 9 & 4 2 & 4 & 5 & 4 & 2 end{bmatrix}]
- Find the intensity gradient of the image. For this, we follow a procedure analogous to Sobel:
- Apply a pair of convolution masks (in (x) and (y) directions:
[G_{x} = begin{bmatrix} -1 & 0 & +1 -2 & 0 & +2 -1 & 0 & +1 end{bmatrix}]
[G_{y} = begin{bmatrix} -1 & -2 & -1 0 & 0 & 0 +1 & +2 & +1 end{bmatrix}]
- Find the gradient strength and direction with:
[begin{array}{l} G = sqrt{ G_{x}^{2} + G_{y}^{2} } theta = arctan(dfrac{ G_{y} }{ G_{x} }) end{array}]
The direction is rounded to one of four possible angles (namely 0, 45, 90 or 135)
- Apply a pair of convolution masks (in (x) and (y) directions:
- Non-maximum suppression is applied. This removes pixels that are not considered to be part of an edge. Hence, only thin lines (candidate edges) will remain.
Hysteresis: The final step. Canny does use two thresholds (upper and lower):
- If a pixel gradient is higher than the upper threshold, the pixel is accepted as an edge
- If a pixel gradient value is below the lower threshold, then it is rejected.
- If the pixel gradient is between the two thresholds, then it will be accepted only if it is connected to a pixel that is above the upper threshold.
Canny recommended a upper:lower ratio between 2:1 and 3:1.
- For more details, you can always consult your favorite Computer Vision book.
Code
Parking Slot Detection Opencv Download
- The tutorial code's is shown lines below. You can also download it from here#include 'opencv2/imgproc.hpp'#include <iostream>using namespace cv;Mat src, src_gray;constint max_lowThreshold = 100;constint kernel_size = 3;{Canny( detected_edges, detected_edges, lowThreshold, lowThreshold*ratio, kernel_size );dst = Scalar::all(0);src.copyTo( dst, detected_edges);imshow( window_name, dst );int main( int argc, char** argv )CommandLineParser parser( argc, argv, '{@input fruits.jpg input image}' );src = imread( samples::findFile( parser.get<String>( '@input' ) ), IMREAD_COLOR ); // Load an imageif( src.empty() )std::cout << 'Could not open or find the image!n' << std::endl;std::cout << 'Usage: ' << argv[0] << ' <Input image>' << std::endl;}dst.create( src.size(), src.type() );cvtColor( src, src_gray, COLOR_BGR2GRAY );namedWindow( window_name, WINDOW_AUTOSIZE );createTrackbar( 'Min Threshold:', window_name, &lowThreshold, max_lowThreshold, CannyThreshold );CannyThreshold(0, 0);waitKey(0);return 0;
- The tutorial code's is shown lines below. You can also download it from hereimport java.awt.Container;import javax.swing.ImageIcon;import javax.swing.JLabel;import javax.swing.JSlider;import javax.swing.event.ChangeListener;import org.opencv.core.Core;import org.opencv.core.Mat;import org.opencv.core.Size;import org.opencv.imgcodecs.Imgcodecs;privatestaticfinalint MAX_LOW_THRESHOLD = 100;privatestaticfinalint KERNEL_SIZE = 3;privateint lowThresh = 0;private Mat srcBlur = new Mat();private Mat dst = new Mat();private JLabel imgLabel;public CannyDetectorDemo(String[] args) {String imagePath = args.length > 0 ? args[0] : '../data/fruits.jpg';if (src.empty()) {System.exit(0);frame = new JFrame('Edge Map (Canny detector demo)');frame.setDefaultCloseOperation(JFrame.EXIT_ON_CLOSE);Image img = HighGui.toBufferedImage(src);addComponentsToPane(frame.getContentPane(), img);// Use the content pane's default BorderLayout. No need for// Display the window.frame.setVisible(true);privatevoid addComponentsToPane(Container pane, Image img) {if (!(pane.getLayout() instanceof BorderLayout)) {pane.add(new JLabel('Container doesn't use BorderLayout!'));}JPanel sliderPanel = new JPanel();sliderPanel.setLayout(new BoxLayout(sliderPanel, BoxLayout.PAGE_AXIS));sliderPanel.add(new JLabel('Min Threshold:'));JSlider slider = new JSlider(0, MAX_LOW_THRESHOLD, 0);slider.setMinorTickSpacing(5);slider.setPaintLabels(true);@OverrideJSlider source = (JSlider) e.getSource();update();});imgLabel = new JLabel(new ImageIcon(img));}privatevoid update() {Imgproc.Canny(srcBlur, detectedEdges, lowThresh, lowThresh * RATIO, KERNEL_SIZE, false);dst = new Mat(src.size(), CvType.CV_8UC3, Scalar.all(0));Image img = HighGui.toBufferedImage(dst);frame.repaint();// Load the native OpenCV library// creating and showing this application's GUI.javax.swing.SwingUtilities.invokeLater(new Runnable() {publicvoid run() {}}
Opencv Defect Detection
- The tutorial code's is shown lines below. You can also download it from hereimport cv2 as cvwindow_name = 'Edge Map'ratio = 3low_threshold = valdetected_edges = cv.Canny(img_blur, low_threshold, low_threshold*ratio, kernel_size)dst = src * (mask[:,:,None].astype(src.dtype))parser = argparse.ArgumentParser(description='Code for Canny Edge Detector tutorial.')parser.add_argument('--input', help='Path to input image.', default='fruits.jpg')if src isNone:print('Could not open or find the image: ', args.input)cv.createTrackbar(title_trackbar, window_name , 0, max_lowThreshold, CannyThreshold)CannyThreshold(0)
- Asks the user to enter a numerical value to set the lower threshold for our Canny Edge Detector (by means of a Trackbar).
- Applies the Canny Detector and generates a mask (bright lines representing the edges on a black background).
- Applies the mask obtained on the original image and display it in a window.
Explanation (C++ code)
Parking Slot Detection Opencv Tool
- Create some needed variables: Note the following:Mat dst, detected_edges;int lowThreshold = 0;constint ratio = 3;constchar* window_name = 'Edge Map';
- We establish a ratio of lower:upper threshold of 3:1 (with the variable ratio).
- We set the kernel size of (3) (for the Sobel operations to be performed internally by the Canny function).
- We set a maximum value for the lower Threshold of (100).
- Loads the source image: CommandLineParser parser( argc, argv, '{@input fruits.jpg input image}' );src = imread( samples::findFile( parser.get<String>( '@input' ) ), IMREAD_COLOR ); // Load an imageif( src.empty() )std::cout << 'Could not open or find the image!n' << std::endl;std::cout << 'Usage: ' << argv[0] << ' <Input image>' << std::endl;}
- Create a matrix of the same type and size of src (to be dst):
- Convert the image to grayscale (using the function cv::cvtColor ):
- Create a window to display the results:
- Create a Trackbar for the user to enter the lower threshold for our Canny detector: createTrackbar( 'Min Threshold:', window_name, &lowThreshold, max_lowThreshold, CannyThreshold );Observe the following:
- The variable to be controlled by the Trackbar is lowThreshold with a limit of max_lowThreshold (which we set to 100 previously)
- Each time the Trackbar registers an action, the callback function CannyThreshold will be invoked.
- Let's check the CannyThreshold function, step by step:
- First, we blur the image with a filter of kernel size 3:
- Second, we apply the OpenCV function cv::Canny : Canny( detected_edges, detected_edges, lowThreshold, lowThreshold*ratio, kernel_size );where the arguments are:
- detected_edges: Source image, grayscale
- detected_edges: Output of the detector (can be the same as the input)
- lowThreshold: The value entered by the user moving the Trackbar
- highThreshold: Set in the program as three times the lower threshold (following Canny's recommendation)
- kernel_size: We defined it to be 3 (the size of the Sobel kernel to be used internally)
- We fill a dst image with zeros (meaning the image is completely black).
- Finally, we will use the function cv::Mat::copyTo to map only the areas of the image that are identified as edges (on a black background). cv::Mat::copyTo copy the src image onto dst. However, it will only copy the pixels in the locations where they have non-zero values. Since the output of the Canny detector is the edge contours on a black background, the resulting dst will be black in all the area but the detected edges.
- We display our result:
Result

After compiling the code above, we can run it giving as argument the path to an image. For example, using as an input the following image:
Moving the slider, trying different threshold, we obtain the following result:
- Notice how the image is superposed to the black background on the edge regions.